Asking Questions So That They Will Be Answered
Asking Questions So That They Will Be Answered
Chu Keong Lee, Qian Wu, Chei Sian Lee and Dion Hoe-Lian Goh
The World Wide Web has enabled knowledge sharing on a global scale. Through groups known as social question and answer (Q&A) communities, people can ask questions and collaboratively co-create knowledge. The question the study investigates is simple: Are the interactions resulting from the questions posted influenced by how the question is framed?
In this research, a hundred questions with the most votes (and generating a total of 14,599 posts) were studied. Eight characteristics of questions, or question cues were investigated: (1) complexity, related to the level of knowledge required to answer the question; (2) specificity, related to how much specialized details are provided in the question; (3) rewards, related to the amount of “bounty” tied to solving the problem; (4) emotional expressiveness, related to the display of personal emotions; (5) self-disclosure, related to the amount of personal information and experienced revealed by the questioner; (6) collaborative climate, related to the socioemotional used to appeal for collaboration; (7) politeness, the use of polite terms; and (8) popularity, related to whether or not the question is tagged with popular keywords.
—How one asks questions matters—
Further, the authors looked into two broad categories of questions, those related to STEM (Science, Technology, Engineering, and Mathematics) and non-STEM. For STEM, the questions were restricted to mathematics, the common link between the different STEM subject areas, and for non-STEM, to the English Language, proficiency of which is critical for success in many non-STEM English subjects.
In social Q&A, the outcomes of knowledge co-creation happens at two levels. The person asking the question benefits as he/she can gain from the knowledge of others. However, the individual is not the only party that benefits. The community benefits in several ways as well: members of the community are able to learn by looking through the posts, members are able to identify the experts in the different subject areas, community bonds which are reciprocity expectations are also strengthened, and a bank of questions and answers is being built up for future reference. In this research, the network configuration of the replies to the questions was thus also examined.
The one hundred most popular questions from each category were collected from StackExchange from 30 September 2010 to 30 September 2019 using its application programming interface (API). Content analysis was conducted to identify the question cues using a coding scheme adapted from previous studies. Four coders were recruited—two with Bachelor’s degrees related to mathematics, and the other two with Bachelor’s degrees related to the English language. The coders were trained with ten questions and coded the remaining 90 questions independently.
Finally, social network analysis was conducted to characterise the response network, a network that illustrates the interactions between questions and responses. This comprised the central node which represented the question, nodes directly connected to the central node which represented the answers and comments posted in response to the central question, and nodes connected to the responses (refer to Figure 1). The node size was used to denote the number of responses it attracted. Larger nodes denoted questions which had more responses than others.
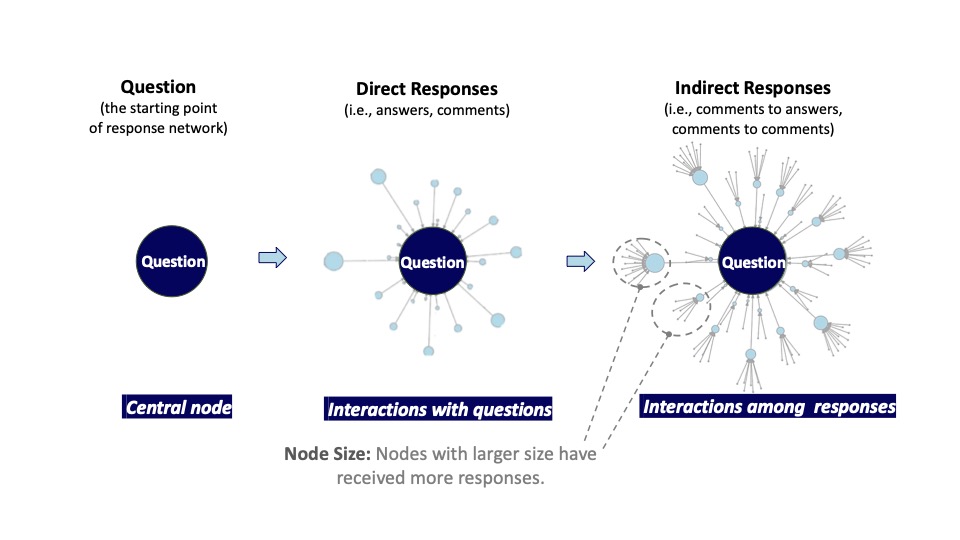
Two types of interactions were analyzed using the igraph R package—direct interaction with questions (measured using degree centrality) and interactions among responses (measured using eigenvector centrality). SPSS was used to compare the influence of the difference goal frames using two-way ANOVA.
The analysis showed that there was a huge difference between the number of posts associated with the top one hundred questions on mathematics (which attracted 9,181 posts) and the English language (which attracted 5,218 posts). This may indicate the level of help that community members were willing to extend to other community members. This difference is surprising because as Hirsch (1996) argues, in mathematics “each question, provided it is phrased unambiguously, has a definite answer.” We would expect that the “preciseness” of mathematics would lead to less discussions.
The percentages of question cues are shown in Figure 2. Lindenberg (2007) suggests that goals “frame” the way people process information and act upon it. In this research, gain goal-frames are those which increase personal resources, hedonic goal-frames are those that focus on improving personal feelings, and normative goal-frames are those that benefit the community. Questions on mathematics tended to use question cues that emphasised gain goals while those on the English language tended to use hedonic and normative goals. Question cues that stressed hedonic and normative goals attracted more responses in both mathematics and English language.
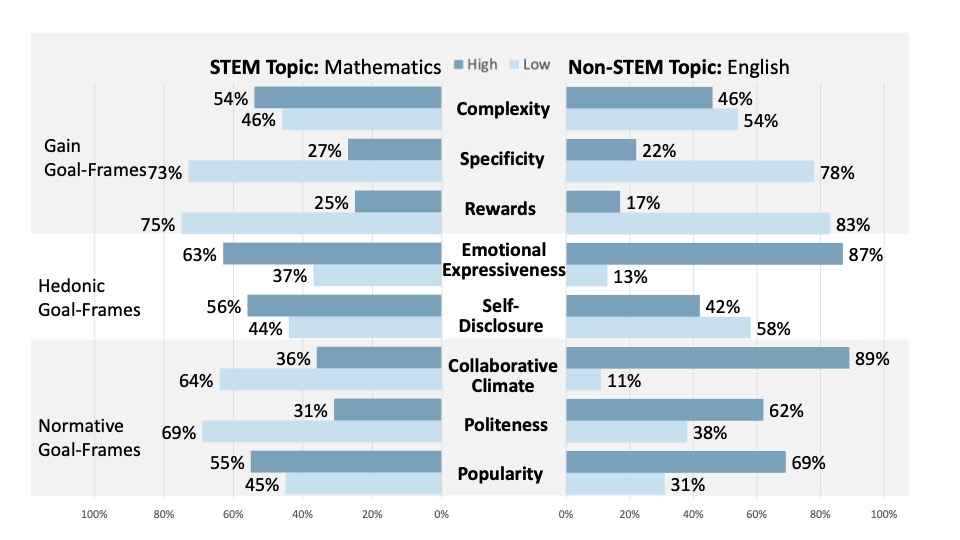
The response networks showed that, for mathematics, there were fewer responses to questions with high specificity and rewards. Moreover, most responses directly connected with the questions. The reverse was true for the English language. High specificity and rewards decreased direct interactions with questions. Questions with high emotional expressiveness attracted more indirect responses, with extensive interactions among the responses. A high collaborative climate attracted many response-to-response connections. In contrast, low popularity generated more interactions among responses.
This research shows that how one asks questions matters! The way a question is posted can get a vibrant discussion going, or not. Questions that provided details and offer rewards have a potential to increase a poster’s prestige, and this increases interaction with questions. However, a high amount of specialised information may decrease the interaction among responses if there are few specialists in the community.
Complexity may not necessarily influence interactions. This may be due to the differences in responders’ interpretation of goals, and the relative nature of complexity. A mathematics question requiring post-secondary knowledge will be difficult for primary school students, but easy for responders with a post-graduate degree.
Questions with hedonic and normative goal frames influence interactions among responses, but not interactions with the questions. Surprisingly, politeness does not influence the response networks. This could be because in academic communities, informational goals are more important that social ones.
This research provides guidance on how to increase the odds of getting one’s questions answered in a social Q&A. For STEM topics, gain goal-frames with high specificity and rewards should be used. In contrast, for non-STEM topics, normative goal-frames would be more useful. If concise and informative responses are preferred, expressions with low emotional expressiveness should be used. Conversely, questions with high emotional expressiveness would invite discussions. In short—how you phrase your questions determine what sort of responses you get.
The original article
Wu, Q., Lee, C. S., & Goh, D. H.-L. (2023). Understanding user-generated questions in social Q&A: A goal-framing approach. Journal of the Association for Information Science and Technology, 74(8), 990–1009. https://doi.org/10.1002/asi.24770
References
Hirsch, R. (1996). Is mathematics a pure science? Science & Society, 60(1), 58-79.
Lindenberg, S., & Steg, L. (2007). Normative, gain and hedonic goal frames guiding environmental behavior. Journal of Social Issues, 63(1), 117-137.
Cite this article in APA as: Lee, C. K., Wu, Q., Lee, C. S. & Goh, D. H. Asking questions so that they will be answered. (2023, August 2). Information Matters, Vol. 3, Issue 8. https://informationmatters.org/2023/07/asking-questions-so-that-they-will-be-answered/
Author
-
Qian Wu is a PhD candidate from Wee Kim Wee School of Communication and Information, Nanyang Technological University, Singapore. Her PhD research focuses on utilizing question cues to manage online knowledge co-creation. More broadly, her research focuses on how visual and linguistic cues can influence human-computer interaction, especially the interactions with conversational AI.