How to Mine Business Value from Bullet Screen Comments in TV Series by Aspect Sentiment Analysis?
How to Mine Business Value from Bullet Screen Comments in TV Series by Aspect Sentiment Analysis?
Jiayue Liu, Ziyao Zhou, Ming Gao, Jiafu Tang, and Weiguo Fan
The rapid growth of online video in recent years has given rise to new and interesting ways for audience to enhance their immersion and viewing experience, such as bullet screen comments (BSCs). BSCs originated from the Japanese video-sharing website NicoNico and are also referred to as “barrages” due to the numerous, short, fast-moving comments scrolling across the screen that resemble a stream of bullets.
—Bullet screen comments (BSCs) are user-generated short comments that express audience opinions and emotions about different aspects of the ongoing video—
BSCs are user-generated short comments that appear as real-time overlays on many video platforms (see Figure 1), expressing the audience opinions and emotions about different aspects of the ongoing video. Unlike traditional long comments after a show, BSCs are often incomplete, ambiguous in context, and correlated over time. Current studies in sentiment analysis of BSCs rarely address these challenges and mine business value, motivating us to develop an aspect-level sentiment analysis framework and explore downstream tasks.
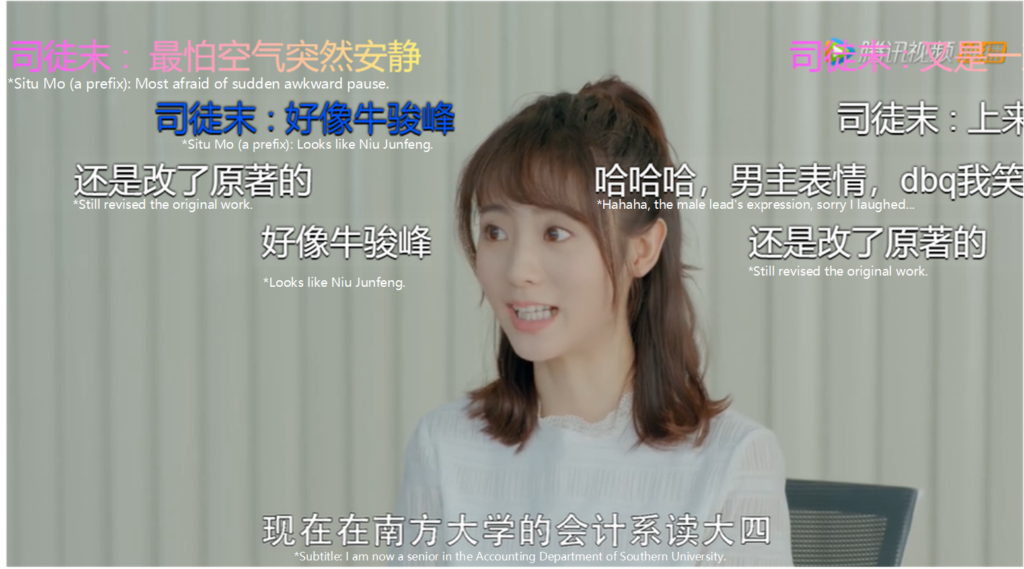
Figure 1. BSCs in a TV-series
The solution we give for the BSCs sentiment classification problem consists of the following steps:
- Based on the collected TV series BSC datasets, we designed a general-purpose fine-grained classification hierarchy that combines aspects and sentimental polarities to recognize the sentiment or aspects of BSCs.
- We established an aspect-level sentiment analysis framework, BSCNET, consisting of a pre-trained language encoder-based deep neural classifier, a novel method of constructing neighbor context for contextual learning of BSCs and semi-supervised learning, aims to enhance semantic understanding and reduce labeling costs (see Figure 2).
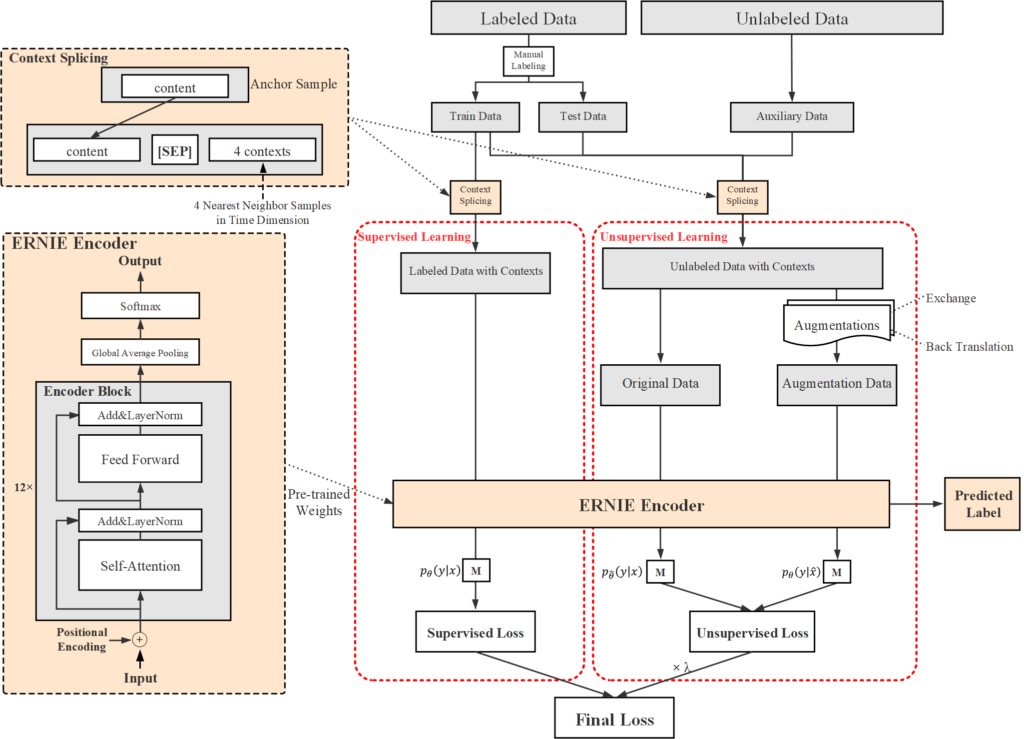
We propose two downstream tasks to validate the practical effects of mining BSCs for business:
- identification of noisy BSCs, which can be used to filter out meaningless BSCs and thus improve the viewing experience of audience.
- the popularity prediction of future episode, which is modeled as the multivariate time series prediction and valuable for optimizing forthcoming content.
The former reveals that fine to coarse-grained fine-tuning can effectively addresses the issue of categorical imbalance when applied to compatible downstream tasks. The latter demonstrates the mediate results of aspect-level sentiment classification predicted by iteratively fine-tune the BSCNET based on history episodes according to the TV series update order. With the increase in the number of BSCs revealed, the performance shows an upward trend. Historical popularity does not always play an important role, so sentiment features and descriptive features are both necessary, while the total weight and ranking of sentiment features are significantly higher. In different TV series, the sentiment features such as non-plot/plot and positive/negative contribute to the prediction differently. Furthermore, our analysis of feature importance reveals an interesting insight: noisy BSCs even play an important role in popularity prediction, which has never been reported before. This finding has encouraged us to develop more powerful tools, from a time series perspective, to analyze audience behavior, assess content attractiveness, and mine valuable insights from BSCs.
In general, our framework provides a solid methodology reference and trade-off options for advanced and in-depth mining of BSCs, and is also generalizable to other short-text classification tasks, particularly in domains with a small corpus or imbalanced categories. Furthermore, we have developed two novel downstream tasks to evaluate the practical effects and performance gains of the pre-trained BSCNET for mining BSCs and explore the potential value of BSCs.
Undoubtedly, more efforts are needed to push the research into a broader field in terms of video BSCs, including multi-genre TV series, movies, short live streams, and educational videos. We urge future researchers to so.
Our recent paper, “Aspect Sentiment Mining of Short Bullet Screen Comments from Online TV Series” (https://doi.org/10.1002/asi.24800) has been published in JASIST.
Cite this article in APA as: Liu, J., Zhou, Z., Gao, M., Tang, J., & Fan, W. (2023, May 23). How to mine business value from bullet screen comments in tv series by aspect sentiment analysis? Information Matters, Vol. 3, Issue 5. https://informationmatters.org/2023/05/how-to-mine-business-value-from-bullet-screen-comments-in-tv-series-by-aspect-sentiment-analysis/