View analysis of personal information leakage and privacy protection in big data era—based on Q method
View Analysis of Personal Information Leakage and Privacy Protection in Big Data Era—Based on Q Method
Lei Huang, City University of Hong Kong
Jingyi Zhou, Wuhan University
Jiecong Lin, City University of Hong Kong
Shengli Deng, Wuhan University
Have you ever been anxious about the exposure of information and encountered a personal information leakage incident? In the current era of big data, people face the risk of personal information leakage while reaping the benefits of big data technologies. People’s attitudes towards personal information privacy are varied and complicated. Some people are conservative to the disclosure to personal information while others hold positive attitudes towards to information disclosure for customized services. Although we can have prior hypothesis about peoples’ views of personal information leakage and privacy protection, the specific types of the views and the reasons behind are not clear.
To investigate the subjective patterns of privacy leakage and protection, we propose a semi-qualitative method-based framework (Q method) that combines the strengths of qualitative and quantitative methods to uncover the key factors underlying people’s views. Furthermore, we utilize Latent Dirichlet Allocation (LDA), TextRank to capture statements data from large-scale literature, app reviews, typical cases, and survey interviews in addition to those from the correspondents.
—People face the risk of personal information leakage while reaping the benefits of big data technologies.—
What is the semi-qualitative method (Q method )?
Specifically, we employ Q methodology to conduct view analysis. Q methodology is a semi-qualitative approach designed to identify typologies of perspectives. It focuses on respondents’ attitudes towards specific issues and seeks to reveal their real opinions, which reflect their subjective patterns. In the Q method, respondents sort and rank statements based on their gradient from “most like how I think” to “least like how I think.” The Q method’s most significant advantage is that it enables respondents to express their actual views because the statements are derived from daily communication, and respondents can evaluate them based on their own criteria. Respondents define themselves through sorting and ranking statements rather than being placed into predefined hypotheses by researchers. Additionally, the statements are not structured based on prior theories or hypotheses, which makes the sample of statements in a Q-study more naturalistic and unstructured, resulting in a more realistic representation of the participants’ views. This reflects the qualitative nature of the Q method. Finally, we employ Principal Component Analysis (PCA) to identify the important factors. This step is quantitative. In summary, the Q method consists of four steps: (1) statement collection, (2) Q sampling, (3) P sampling, (4) Q sorting, and (5) PCA analysis.
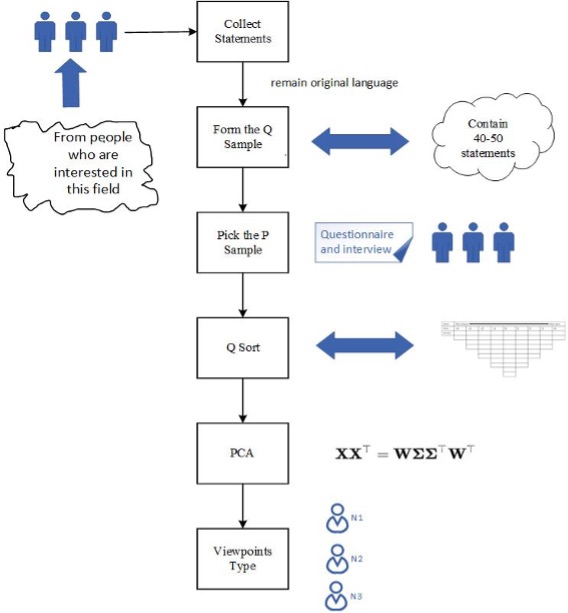
How we adapt and improve Q mthod for view analysis of information leakage?
The first step is to collect statements. To obtain a comprehensive understanding of users’ viewpoints, this study uses Latent Dirichlet Allocation (LDA), TextRank, and other information extraction technologies to capture statements from large-scale literature, app reviews, typical cases, and survey interviews, which can be considered as additional resources for viewpoints, in addition to the statements from the respondents themselves. The former method clusters the statements, and the latter ranks them. Finally, we obtain 50 statements as Q samples.
The third step is the collection of the P sample, which involves selecting respondents. In the process of collecting Q sorting, questionnaires were used to collect the basic personal information of the respondents, and invalid samples were excluded. We selected 30 respondents interested in big data technology and concerned about personal information protection and privacy leakage through pre-interviews. They were then asked to perform Q ranking by the questionnaire method. Respondents sorted their statements based on our preset normal distribution structure. Respondents classified the 50 statements into 9 categories based on their relative agreement.
This study employs the PQmethod, which incorporates the PCA method for data processing and analysis. The primary process includes five steps: statement entry, related data setting, Q sorting input, factor analysis, actor rotation, and calculating the factor score. Preliminary analysis results can be obtained by applying PQmethod for factor analysis. Respondents of different groups are analyzed in combination with the actual situation.
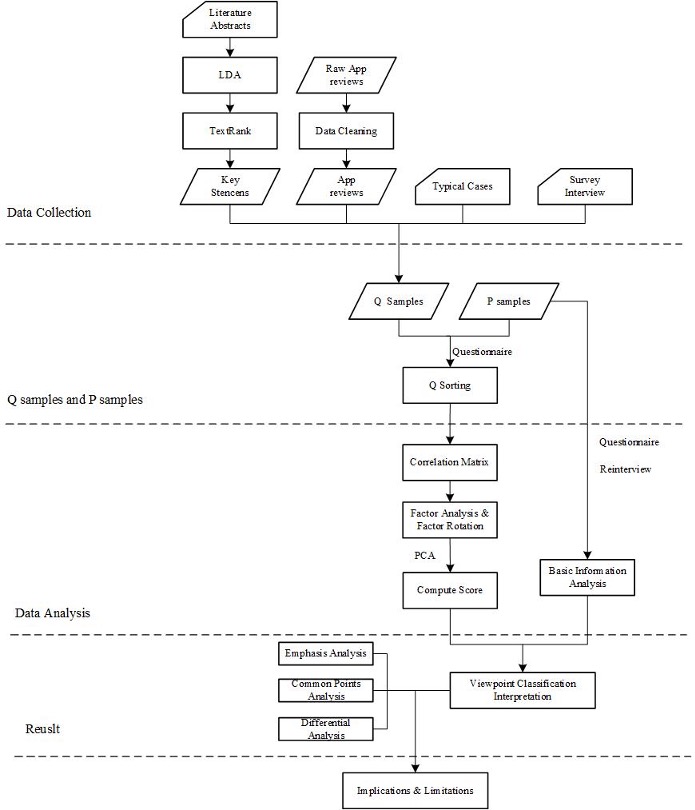
What are the subjective patterns of information disclosure of the respondents?
By adopting the Q method that aims for studying subjective thought patterns to identify users’ potential views, we have identified three categories of stakeholders’ subjectivities: macro-policy sensitive, trade-off and personal information sensitive, each of which perceives different risk and affordance of information leakage and importance and urgency of privacy protection. Macro-policy sensitive users are likely to focus on the policy related to privacy protection especially the changes that policy should make in response to big data technology. Trade-off users are willing to disclose personal information in exchange for benefits like personalized service. Personal information sensitive users pay top attention to the use of personal information and have the lowest level of acceptance for information disclosure. All of them share the consensus that personal information should be protected in any use stage but they hold different attitudes toward privacy disclosure in specific fields like finance and medicine due to their personal experiences. All of the subjectivities of the respondents reflect the awareness of the issue of information leakage, that is, the interested parties like social network sites are unable to protect their full personal information while reflecting varied resistance and susceptibility of disclosing personal information for big data technology applications.
What insights can be derived from the subjective patterns to direct the personal information protection
The subjective patterns we identified provide the basis for whether the integrate, extend and special use of personal data is embraced or rejected by the groups with related interests. In particular, the online service providers including the relevant departments of government and companies should offer privacy assurance mechanisms to help users control their data appropriately. To make sure the mechanism can protect the user private information effectively and accurately, governments and corporations should strengthen the role of privacy protection because all users’ subjective views expressed more or less distrust of existing institutions in terms of privacy protection. From the policy perspective, the governments should pay attention to the definition of privacy since there are heterogeneities in views of users on what information they are not want to disclose.
The article upon which this translation is based is available at: https://doi.org/10.1108/AJIM-05-2021-0144
Cite this article in APA as: Huang, L., Zhou, J., Lin, J. & Deng, S. (2023, March 1). View analysis of personal information leakage and privacy protection in big data era—based on Q method. Information Matters, Vol. 3, Issue 3. https://informationmatters.org/2023/03/view-analysis-of-personal-information-leakage-and-privacy-protection-in-big-data-era-based-on-q-method/
Author
-
I’m a third-year Ph.D. student of the Department of Computer Science, City University of Hong Kong. My research interests include BioNLP and Machine learning in drug development.
View all posts