Lost at starting line: who will not be able to adapt to university life?
Lost at the Starting Line: Who Will Not Be Able to Adapt to University Life?
Teng Guo
Motivation
Higher education plays a significant role in education as it imparts professional skills that help students facilitate a smooth transition into the workforce market. However, the transition from secondary education to higher education could be challenging for most freshmen due to a completely unknown environment and autonomous learning style, which leads to the emergence of the maladaptation phenomenon (Bruffaerts et al., 2018) (Figure 1). If universities can’t offer guidance in time, the situation of these students will deteriorate, and some extreme behaviors may occur, such as suicide. Currently, the main method in this field is to detect the status of students based on questionnaires. This method is time- and cost-consuming and difficult to scale questionnaires to large-scale student populations (Bai et al., 2021; Guo et al., 2021). How to predict students with maladaptation in advance based on educational big data is a problem worthy of attention.
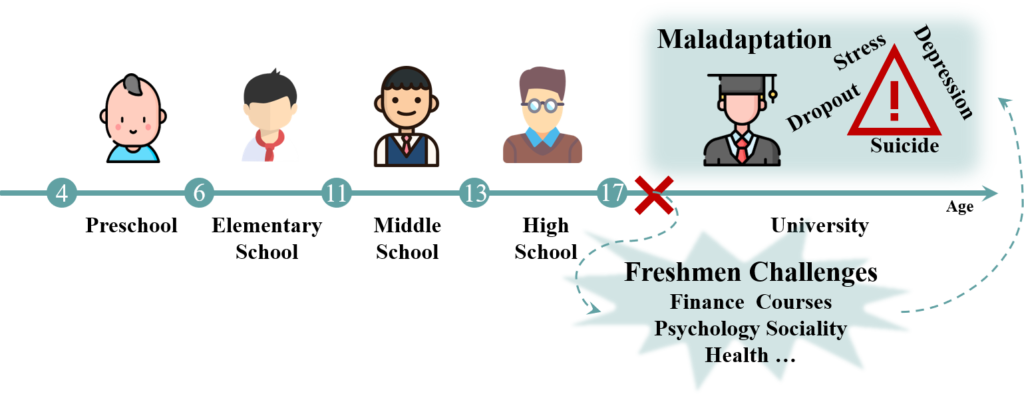
Implementing data-driven prediction of maladaptation is not an easy task. First, maladaptation is difficult to be accurately quantified because it has no typical symptom (Ameri et al., 2016; Moeller et al., 2020). In this case, how to design a suitable indicator to indirectly quantify maladaptation is an important challenge in this field. Second, currently, deep learning is the most popular algorithm, but the inexplicability makes it difficult to gain the trust of education stakeholders. Moreover, due to the limitation of educational resources, the classification experiment aimed at maladaptive phenomena cannot provide an effective reference for educational management. For example, if a staff member is responsible for 30 students, and the algorithm tells you that 10 of them will feel maladaptive to university life. Since he did not know the severity of the maladaptive students, he could not know which student needed to be the first to receive the psychological intervention.
—If universities can't offer guidance in time, the situation of these students will deteriorate.—
Methodology
We design a targeted algorithm framework to tackle the above challenges and, the basic ideas are as follows. First, as common sense, academic performance is the most important standard for quantifying students. Therefore, we assume that fluctuations in academic performance at the beginning of university are related to freshmen’s adaptability to university life closely. Specifically, we compare the rankings of the academic performance of freshmen before and after they entered university, and indirectly identify students with maladaptation through changes in rankings. Second, we propose a variation on the Random Forest, named Priority Forest, to calculate the priority of students with maladaptation. Compared with traditional ensemble strategies that ensemble base classifiers through bagging, boosting, and stacking, our algorithm assigns weights to base classifiers by highlighting their inter-individual differences.
Experiments
We collect the personal information of 2634 students from a Chinese university, including psychology features, intelligence features and demographic features. First, we rank students on the performance of the national university entrance examination to represent a student’s academic performance prior to admission. Meanwhile, we rank students on the GPA of the final exam of the first semester to represent the performance of students after admission. We indirectly identify the student with maladaptation according to the difference between the two rankings. Second, we design a variant of the random forest algorithm to predict students with maladaptation. Inspired by the loss function of the learning-to-rank algorithm, we create our loss function to distinguish each two students based on the initial idea that maximizes the difference between two students ranked next to each other. The experimental results demonstrate the effectiveness of the proposed method.
Conclusion
We propose a framework for automatically predicting university freshmen with maladaptation and design experiments to demonstrate the effectiveness of the proposed framework. All the data comes from the learning management system which avoids manual data collection, and achieves the data-driven prediction of university freshmen with maladaptation. Our research not only fills the gap in the field of student maladaptation-related research, but also provides insights into data-driven smart campus construction. In the future, we intend to define the maladaptation phenomenon from various aspects and additionally, we will try to incorporate our framework into a cutting-edge information system of education to detect students’ status in real-time.
The original article on which this essay is based:
Guo, T., Bai, X., Zhen, S., Abid, S., & Xia, F. (2022). Lost at starting line: Predicting maladaptation of university freshmen based on educational big data. Journal of the Association for Information Science and Technology.
References
Bruffaerts, R., Mortier, P., Kiekens, G., Auerbach, R. P., Cuijpers, P., Demyttenaere, K., & Kessler, R. C. (2018). Mental health problems in college freshmen: Prevalence and academic functioning. Journal of affective disorders, 225, 97-103.
Guo, T., Bai, X., Tian, X., Firmin, S., & Xia, F. (2021). Educational anomaly analytics: Features, methods, and challenges. Frontiers in big Data, 4.
Bai, X., Zhang, F., Li, J., Guo, T., Aziz, A., Jin, A., & Xia, F. (2021). Educational big data: predictions, applications and challenges. Big Data Research, 26, 100270.
Ameri, S., Fard, M. J., Chinnam, R. B., and Reddy, C. K. (2016). Survival analysis based framework for early prediction of student dropouts. In Proceedings of the 25th ACM International on Conference on Information and Knowledge Management, pages 903–912. ACM.
Moeller, R. W., Seehuus, M., Simonds, J., Lorton, E., Randle, T. S., Richter, C., & Peisch, V. (2020). The differential role of coping, physical activity, and mindfulness in college student adjustment. Frontiers in Psychology, 11, 1858.
Cite this article in APA as: Guo, T. (2022, December 8). Lost at the starting line: Who will not be able to adapt to university life? Information Matters, Vol. 2, Issue 12. https://informationmatters.org/2022/11/lost-at-starting-line-who-will-not-be-able-to-adapt-to-university-life/