What Should We Teach? A Human-Centered Data Science Graduate Curriculum Model Design for iField Schools
What Should We Teach? A Human-Centered Data Science Graduate Curriculum Model Design for iField Schools
Hao Xu, Dan Wu
Data science education is an important measure to address current vacancies in data scientists. As a discipline with solid multidisciplinary elements, there are numerous approaches to developing data science teaching methods and curricula, with a general emphasis on computation or statistics. As one of the global key forces in data science education, information schools, also known as iField schools, have long respected human values such as privacy and data ethics and have prioritized the development of socially acceptable data science solutions. As a result, current teaching techniques and courses that emphasize computational or mathematical statistics are incompatible with iField schools. Given that graduate programs are the norm in data science education, creating a data science graduate curriculum model that is broadly applicable to information schools, depending on their specific characteristics, is vital.
—Creating a data science graduate curriculum model that is broadly applicable to information schools is vital.—
To achieve these goals, we collected 96 data science graduate programs offered by the iField schools from iSchools members and the well-known data science website “Datascience. Community.” These 96 programs’ details were copied and archived, including nation, school, department, program name, program description, program outcome, course, and course description. We then used a combined research method based on inductive content analysis to examine and identify the competencies and skills development of data science graduate education in the iField schools and the knowledge areas covered by the courses.
According to the findings, the data science graduate programs at iField schools are committed to providing students with three types of data science competencies: professional, practical, and personal, as well as four types of data science skills: programming languages, big data management platforms, operating systems, and domain knowledge. The curriculum of the iField schools’ data science graduate programs covers 12 knowledge topics, including practice and project management, data mining analysis and modeling, library and information science, human and society, data processing storage and management, mathematics and statistics, computer science and artificial intelligence, algorithms and programming, research design and research methods, introduction to data science, big data and big data applications, and human-computer interaction.
Based on the above findings, we reconstructed the 12 curriculum knowledge topics into four major curriculum modules based on the “Humanistic Model” proposed by Weinstein and Fantini in 1970, including (a) data-driven methods and techniques module; (b) domain knowledge module; (c) legal, moral, and ethical data module; and (d) personal trait formation and development module. For each module, specific knowledge topics, case studies, and competency and skill development objectives are provided. With these four modules as the core, a human-centered data science graduate curriculum model is formed.
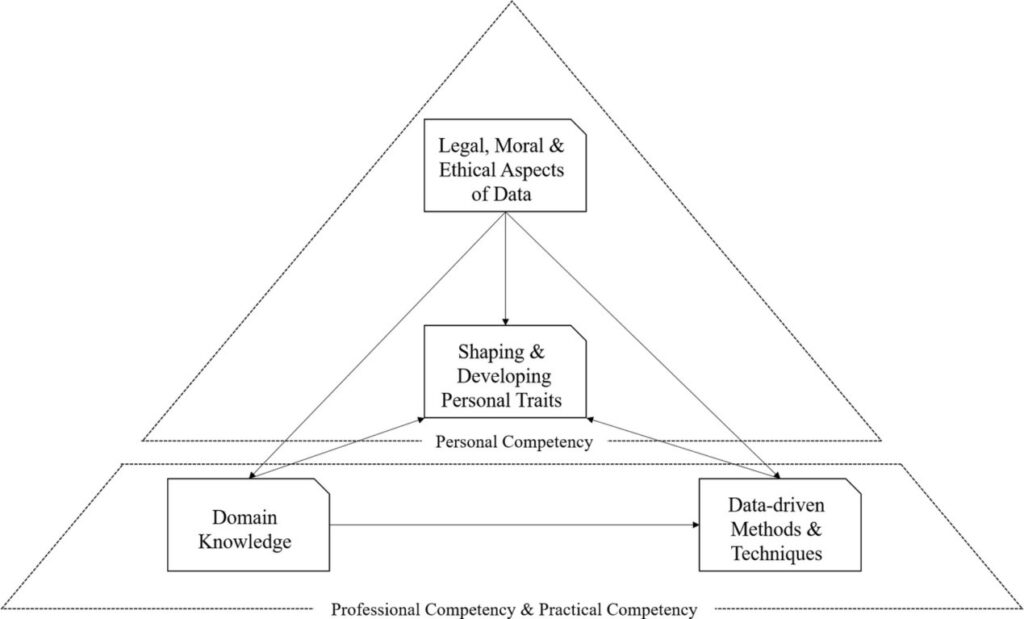
By contrast, the data science curriculum model proposed in this study examines data science through the lens of information science. This curriculum model approaches data science education from a human-centered perspective, takes a holistic view of the data lifecycle, emphasizes the morality of data, and places a premium on its influence on society. The human-centered data science curriculum model strikes an appropriate balance between computational and statistical reasoning, ethical and legal concerns, and individual characteristics. It is important to note that this human-centered data science curriculum model does not mean that students should abandon shaping their computational and statistical thinking. Rather, they should learn advanced analytical languages and data science tools. This is reflected in the curriculum model’s data-driven methods and techniques module. By shaping students’ traits at the core, students will be equipped with sufficient self-learning skills and computational logic to cope with the constant evolution of algorithms and tools. This will allow students to stay on top of their data scientist careers for a long time.
The curriculum model developed in this study provides a universal curriculum development framework for the development of data science graduate education in information schools and lays the foundation for the compatibility and portability of data science graduate education in the iField schools.
Wu, D., Xu, H., Sun, Y., & Lv, S. (2022). What should we teach? A human-centered data science graduate curriculum model design for iField schools. Journal of the Association for Information Science and Technology. https://doi.org/10.1002/asi.24644.
Cite this article in APA as: Xu, H. & Wu, D. (2022, July 13). What should we teach? A human-centered data science graduate curriculum model design for iField schools. Information Matters, Vol. 2, Issue 7. https://informationmatters.org/2022/06/what-should-we-teach-a-human-centered-data-science-graduate-curriculum-model-design-for-ifield-schools/
Authors
-
Hao Xu is a Ph.D. candidate at Wuhan University's School of Information Management. Hao's research interests focus on data science, information behavior, and human-computer interaction.
View all posts -
Dan Wu (Ph.D) is a Professor at Wuhan University, China. Her research interests include information retrieval, information behavior, and human-computer interaction. One of her most important publications is Mobile Search Behaviors: An In-depth Analysis based on Contexts, APPs and Devices, published by Morgan & Claypool.
View all posts